学术海报
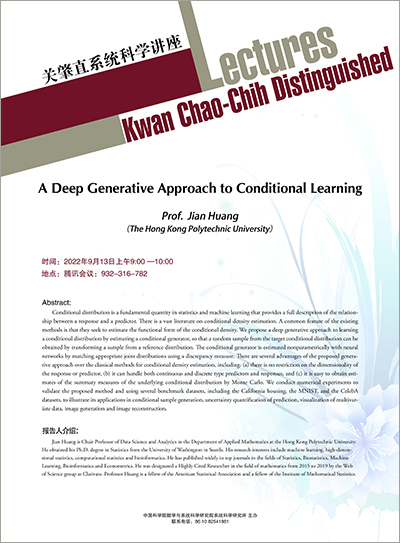
【摘要】
Conditional distribution is a fundamental quantity in statistics and machine learning that provides a full description of the relationship between a response and a predictor. There is a vast literature on conditional density estimation. A common feature of the existing methods is that they seek to estimate the functional form of the conditional density. We propose a deep generative approach to learning a conditional distribution by estimating a conditional generator, so that a random sample from the target conditional distribution can be obtained by transforming a sample from a reference distribution. The conditional generator is estimated nonparametrically with neural networks by matching appropriate joint distributions using a discrepancy measure. There are several advantages of the proposed generative approach over the classical methods for conditional density estimation, including: (a) there is no restriction on the dimensionality of the response or predictor, (b) it can handle both continuous and discrete type predictors and responses, and (c) it is easy to obtain estimates of the summary measures of the underlying conditional distribution by Monte Carlo. We conduct numerical experiments to validate the proposed method and using several benchmark datasets, including the California housing, the MNIST, and the CelebA datasets, to illustrate its applications in conditional sample generation, uncertainty quantification of prediction, visualization of multivariate data, image generation and image reconstruction.
【报告人简介】
Jian Huang is Chair Professor of Data Science and Analytics in the Department of Applied Mathematics at the Hong Kong Polytechnic University. He obtained his Ph.D. degree in Statistics from the University of Washington in Seattle. His research interests include machine learning, high-dimensional statistics, computational statistics and bioinformatics. He has published widely in top journals in the fields of Statistics, Biostatistics, Machine Learning, Bioinformatics and Econometrics. He was designated a Highly Cited Researcher in the field of mathematics from 2015 to 2019 by the Web of Science group at Clarivate. Professor Huang is a fellow of the American Statistical Association and a fellow of the Institute of Mathematical Statistics.